Practical Time Series Analysis. Master Time Series Data Processing, Visualization, and Modeling using Python

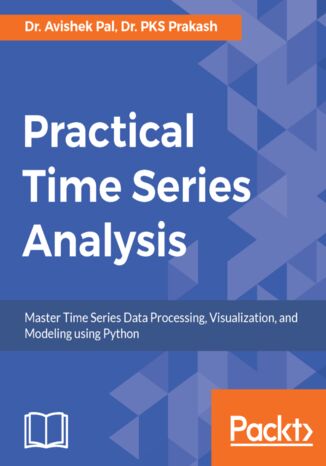
- Wydawnictwo:
- Packt Publishing
- Ocena:
- Stron:
- 244
- Dostępne formaty:
-
PDFePubMobi
Opis
książki
:
Practical Time Series Analysis. Master Time Series Data Processing, Visualization, and Modeling using Python
The book starts with descriptive analysis to create insightful visualizations of internal structures such as trend, seasonality, and autocorrelation. Next, the statistical methods of dealing with autocorrelation and non-stationary time series are described. This is followed by exponential smoothing to produce meaningful insights from noisy time series data. At this point, we shift focus towards predictive analysis and introduce autoregressive models such as ARMA and ARIMA for time series forecasting. Later, powerful deep learning methods are presented, to develop accurate forecasting models for complex time series, and under the availability of little domain knowledge. All the topics are illustrated with real-life problem scenarios and their solutions by best-practice implementations in Python.
The book concludes with the Appendix, with a brief discussion of programming and solving data science problems using Python.
Wybrane bestsellery
Packt Publishing - inne książki
Dzięki opcji "Druk na żądanie" do sprzedaży wracają tytuły Grupy Helion, które cieszyły sie dużym zainteresowaniem, a których nakład został wyprzedany.
Dla naszych Czytelników wydrukowaliśmy dodatkową pulę egzemplarzy w technice druku cyfrowego.
Co powinieneś wiedzieć o usłudze "Druk na żądanie":
- usługa obejmuje tylko widoczną poniżej listę tytułów, którą na bieżąco aktualizujemy;
- cena książki może być wyższa od początkowej ceny detalicznej, co jest spowodowane kosztami druku cyfrowego (wyższymi niż koszty tradycyjnego druku offsetowego). Obowiązująca cena jest zawsze podawana na stronie WWW książki;
- zawartość książki wraz z dodatkami (płyta CD, DVD) odpowiada jej pierwotnemu wydaniu i jest w pełni komplementarna;
- usługa nie obejmuje książek w kolorze.
Masz pytanie o konkretny tytuł? Napisz do nas: sklep@helion.pl
Książka drukowana

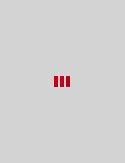
Oceny i opinie klientów: Practical Time Series Analysis. Master Time Series Data Processing, Visualization, and Modeling using Python Avishek Pal, PKS Prakash (0)
Weryfikacja opinii następuję na podstawie historii zamówień na koncie Użytkownika umieszczającego opinię. Użytkownik mógł otrzymać punkty za opublikowanie opinii uprawniające do uzyskania rabatu w ramach Programu Punktowego.