Python Data Cleaning Cookbook. Prepare your data for analysis with pandas, NumPy, Matplotlib, scikit-learn, and OpenAI - Second Edition Michael Walker

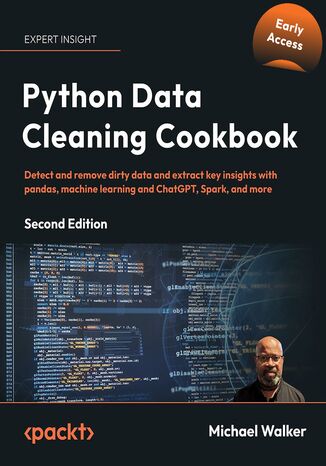
- Autor:
- Michael Walker
- Serie wydawnicze:
- Learning
- Wydawnictwo:
- Packt Publishing
- Ocena:
- Stron:
- 486
- Dostępne formaty:
-
PDFePub
Opis
książki
:
Python Data Cleaning Cookbook. Prepare your data for analysis with pandas, NumPy, Matplotlib, scikit-learn, and OpenAI - Second Edition
Fully updated to the latest version of Python and all relevant tools, this book will teach you how to manipulate and clean data to get it into a useful form. he current edition focuses on advanced techniques like machine learning and AI-specific approaches and tools for data cleaning along with the conventional ones. The book also delves into tips and techniques to process and clean data for ML, AI, and NLP models. You will learn how to filter and summarize data to gain insights and better understand what makes sense and what does not, along with discovering how to operate on data to address the issues you've identified. Next, you’ll cover recipes for using supervised learning and Naive Bayes analysis to identify unexpected values and classification errors and generate visualizations for exploratory data analysis (EDA) to identify unexpected values. Finally, you’ll build functions and classes that you can reuse without modification when you have new data.
By the end of this Data Cleaning book, you'll know how to clean data and diagnose problems within it.
Wybrane bestsellery
Zobacz pozostałe książki z serii Learning
Packt Publishing - inne książki
Dzięki opcji "Druk na żądanie" do sprzedaży wracają tytuły Grupy Helion, które cieszyły sie dużym zainteresowaniem, a których nakład został wyprzedany.
Dla naszych Czytelników wydrukowaliśmy dodatkową pulę egzemplarzy w technice druku cyfrowego.
Co powinieneś wiedzieć o usłudze "Druk na żądanie":
- usługa obejmuje tylko widoczną poniżej listę tytułów, którą na bieżąco aktualizujemy;
- cena książki może być wyższa od początkowej ceny detalicznej, co jest spowodowane kosztami druku cyfrowego (wyższymi niż koszty tradycyjnego druku offsetowego). Obowiązująca cena jest zawsze podawana na stronie WWW książki;
- zawartość książki wraz z dodatkami (płyta CD, DVD) odpowiada jej pierwotnemu wydaniu i jest w pełni komplementarna;
- usługa nie obejmuje książek w kolorze.
Masz pytanie o konkretny tytuł? Napisz do nas: sklep@helion.pl
Książka drukowana

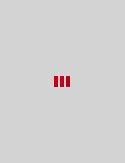
Oceny i opinie klientów: Python Data Cleaning Cookbook. Prepare your data for analysis with pandas, NumPy, Matplotlib, scikit-learn, and OpenAI - Second Edition Michael Walker
(0)