Machine Learning for Time-Series with Python. Use Python to forecast, predict, and detect anomalies with state-of-the-art machine learning methods - Second Edition Ben Auffarth

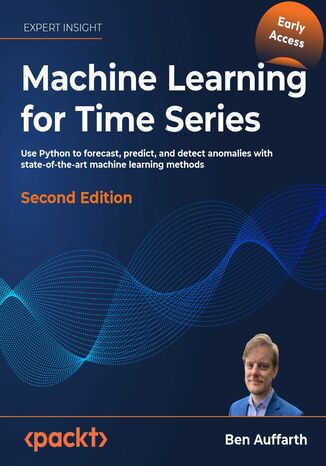
- Autor:
- Ben Auffarth
- Serie wydawnicze:
- Learning
- Wydawnictwo:
- Packt Publishing
- Ocena:
- Dostępny format:
-
ePub
Opis
książki
:
Machine Learning for Time-Series with Python. Use Python to forecast, predict, and detect anomalies with state-of-the-art machine learning methods - Second Edition
This fully updated second edition starts by re-introducing the basics of time series and then helps you get to grips with traditional autoregressive models as well as modern non-parametric models. By observing practical examples and the theory behind them, you will gain a deeper understanding of loading time-series datasets from any source and a variety of models, such as deep learning recurrent neural networks, causal convolutional network models, and gradient boosting with feature engineering. This book will also help you choose the right model for the right problem by explaining the theory behind several useful models. New updates include a chapter on forecasting and extracting signals on financial markets and case studies with relevant examples from operations management, digital marketing, and healthcare.
By the end of this book, you should feel at home with effectively analyzing and applying machine learning methods to time series.
Wybrane bestsellery
Ben Auffarth - pozostałe książki
Zobacz pozostałe książki z serii Learning
Packt Publishing - inne książki
Dzięki opcji "Druk na żądanie" do sprzedaży wracają tytuły Grupy Helion, które cieszyły sie dużym zainteresowaniem, a których nakład został wyprzedany.
Dla naszych Czytelników wydrukowaliśmy dodatkową pulę egzemplarzy w technice druku cyfrowego.
Co powinieneś wiedzieć o usłudze "Druk na żądanie":
- usługa obejmuje tylko widoczną poniżej listę tytułów, którą na bieżąco aktualizujemy;
- cena książki może być wyższa od początkowej ceny detalicznej, co jest spowodowane kosztami druku cyfrowego (wyższymi niż koszty tradycyjnego druku offsetowego). Obowiązująca cena jest zawsze podawana na stronie WWW książki;
- zawartość książki wraz z dodatkami (płyta CD, DVD) odpowiada jej pierwotnemu wydaniu i jest w pełni komplementarna;
- usługa nie obejmuje książek w kolorze.
Masz pytanie o konkretny tytuł? Napisz do nas: sklep@helion.pl
Książka drukowana

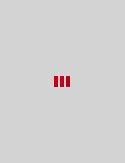
Oceny i opinie klientów: Machine Learning for Time-Series with Python. Use Python to forecast, predict, and detect anomalies with state-of-the-art machine learning methods - Second Edition Ben Auffarth
(0)