Applied Deep Learning on Graphs. Leveraging Graph Data to Generate Impact Using Specialized Deep Learning Architectures

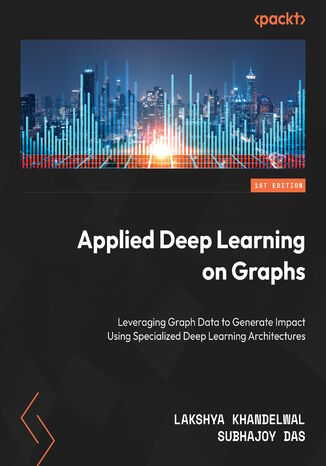
- Serie wydawnicze:
- Hands-on
- Wydawnictwo:
- Packt Publishing
- Ocena:
Opis
książki
:
Applied Deep Learning on Graphs. Leveraging Graph Data to Generate Impact Using Specialized Deep Learning Architectures
By the end of this book, readers master both underlying ideas and hands-on coding skills on real-world use cases and examples along the way. Readers grasp not just how to effectively leverage graph neural networks today but also the promising frontiers to influence where the field may evolve next.
Wybrane bestsellery
Zobacz pozostałe książki z serii Hands-on
Packt Publishing - inne książki
Dzięki opcji "Druk na żądanie" do sprzedaży wracają tytuły Grupy Helion, które cieszyły sie dużym zainteresowaniem, a których nakład został wyprzedany.
Dla naszych Czytelników wydrukowaliśmy dodatkową pulę egzemplarzy w technice druku cyfrowego.
Co powinieneś wiedzieć o usłudze "Druk na żądanie":
- usługa obejmuje tylko widoczną poniżej listę tytułów, którą na bieżąco aktualizujemy;
- cena książki może być wyższa od początkowej ceny detalicznej, co jest spowodowane kosztami druku cyfrowego (wyższymi niż koszty tradycyjnego druku offsetowego). Obowiązująca cena jest zawsze podawana na stronie WWW książki;
- zawartość książki wraz z dodatkami (płyta CD, DVD) odpowiada jej pierwotnemu wydaniu i jest w pełni komplementarna;
- usługa nie obejmuje książek w kolorze.
Masz pytanie o konkretny tytuł? Napisz do nas: sklep@helion.pl
Książka drukowana

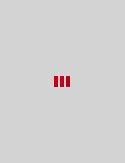
Oceny i opinie klientów: Applied Deep Learning on Graphs. Leveraging Graph Data to Generate Impact Using Specialized Deep Learning Architectures Lakshya Khandelwal, Subhajoy Das (0)
Weryfikacja opinii następuję na podstawie historii zamówień na koncie Użytkownika umieszczającego opinię. Użytkownik mógł otrzymać punkty za opublikowanie opinii uprawniające do uzyskania rabatu w ramach Programu Punktowego.