Data Engineering Best Practices. Architect robust and cost-effective data solutions in the cloud era Richard J. Schiller, David Larochelle

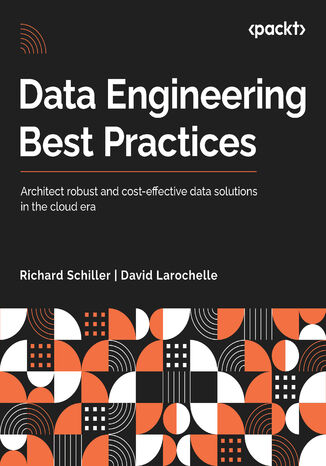
- Autorzy:
- Richard J. Schiller, David Larochelle
- Serie wydawnicze:
- Hands-on
- Wydawnictwo:
- Packt Publishing
- Ocena:
- Stron:
- 550
- Dostępne formaty:
-
PDFePub
Opis
książki
:
Data Engineering Best Practices. Architect robust and cost-effective data solutions in the cloud era
You’ll start by defining the challenges data engineers face and understand how this agile and future-proof comprehensive data solution architecture addresses them. As you explore the extensive toolkit, mastering the capabilities of various instruments, you’ll gain the knowledge needed for independent research. Covering everything you need, right from data engineering fundamentals, the guide uses real-world examples to illustrate potential solutions. It elevates your skills to architect scalable data systems, implement agile development processes, and design cloud-based data pipelines. The book further equips you with the knowledge to harness serverless computing and microservices to build resilient data applications.
By the end, you'll be armed with the expertise to design and deliver high-performance data engineering solutions that are not only robust, efficient, and secure but also future-ready.
Wybrane bestsellery
Zobacz pozostałe książki z serii Hands-on
Packt Publishing - inne książki
Dzięki opcji "Druk na żądanie" do sprzedaży wracają tytuły Grupy Helion, które cieszyły sie dużym zainteresowaniem, a których nakład został wyprzedany.
Dla naszych Czytelników wydrukowaliśmy dodatkową pulę egzemplarzy w technice druku cyfrowego.
Co powinieneś wiedzieć o usłudze "Druk na żądanie":
- usługa obejmuje tylko widoczną poniżej listę tytułów, którą na bieżąco aktualizujemy;
- cena książki może być wyższa od początkowej ceny detalicznej, co jest spowodowane kosztami druku cyfrowego (wyższymi niż koszty tradycyjnego druku offsetowego). Obowiązująca cena jest zawsze podawana na stronie WWW książki;
- zawartość książki wraz z dodatkami (płyta CD, DVD) odpowiada jej pierwotnemu wydaniu i jest w pełni komplementarna;
- usługa nie obejmuje książek w kolorze.
Masz pytanie o konkretny tytuł? Napisz do nas: sklep@helion.pl
Książka drukowana

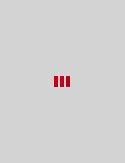
Oceny i opinie klientów: Data Engineering Best Practices. Architect robust and cost-effective data solutions in the cloud era Richard J. Schiller, David Larochelle
(0)