Mastering PyTorch. Create and deploy deep learning models from CNNs to multimodal models, LLMs, and beyond - Second Edition Ashish Ranjan Jha

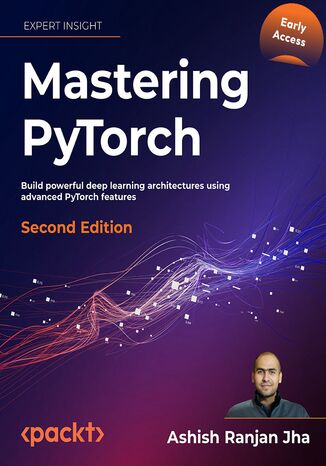
- Autor:
- Ashish Ranjan Jha
- Serie wydawnicze:
- Learning
- Wydawnictwo:
- Packt Publishing
- Ocena:
- Stron:
- 558
- Dostępne formaty:
-
PDFePub
Opis
książki
:
Mastering PyTorch. Create and deploy deep learning models from CNNs to multimodal models, LLMs, and beyond - Second Edition
You’ll build convolutional neural networks for image classification and recurrent neural networks and transformers for sentiment analysis. As you advance, you'll apply deep learning across different domains, such as music, text, and image generation, using generative models, including diffusion models. You'll not only build and train your own deep reinforcement learning models in PyTorch but also learn to optimize model training using multiple CPUs, GPUs, and mixed-precision training. You’ll deploy PyTorch models to production, including mobile devices. Finally, you’ll discover the PyTorch ecosystem and its rich set of libraries. These libraries will add another set of tools to your deep learning toolbelt, teaching you how to use fastai to prototype models and PyTorch Lightning to train models. You’ll discover libraries for AutoML and explainable AI (XAI), create recommendation systems, and build language and vision transformers with Hugging Face.
By the end of this book, you'll be able to perform complex deep learning tasks using PyTorch to build smart artificial intelligence models.
Wybrane bestsellery
Zobacz pozostałe książki z serii Learning
Packt Publishing - inne książki
Dzięki opcji "Druk na żądanie" do sprzedaży wracają tytuły Grupy Helion, które cieszyły sie dużym zainteresowaniem, a których nakład został wyprzedany.
Dla naszych Czytelników wydrukowaliśmy dodatkową pulę egzemplarzy w technice druku cyfrowego.
Co powinieneś wiedzieć o usłudze "Druk na żądanie":
- usługa obejmuje tylko widoczną poniżej listę tytułów, którą na bieżąco aktualizujemy;
- cena książki może być wyższa od początkowej ceny detalicznej, co jest spowodowane kosztami druku cyfrowego (wyższymi niż koszty tradycyjnego druku offsetowego). Obowiązująca cena jest zawsze podawana na stronie WWW książki;
- zawartość książki wraz z dodatkami (płyta CD, DVD) odpowiada jej pierwotnemu wydaniu i jest w pełni komplementarna;
- usługa nie obejmuje książek w kolorze.
Masz pytanie o konkretny tytuł? Napisz do nas: sklep@helion.pl
Książka drukowana

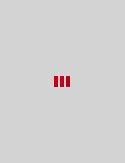
Oceny i opinie klientów: Mastering PyTorch. Create and deploy deep learning models from CNNs to multimodal models, LLMs, and beyond - Second Edition Ashish Ranjan Jha
(0)